The principles of responsible AI and how they apply to AI training data
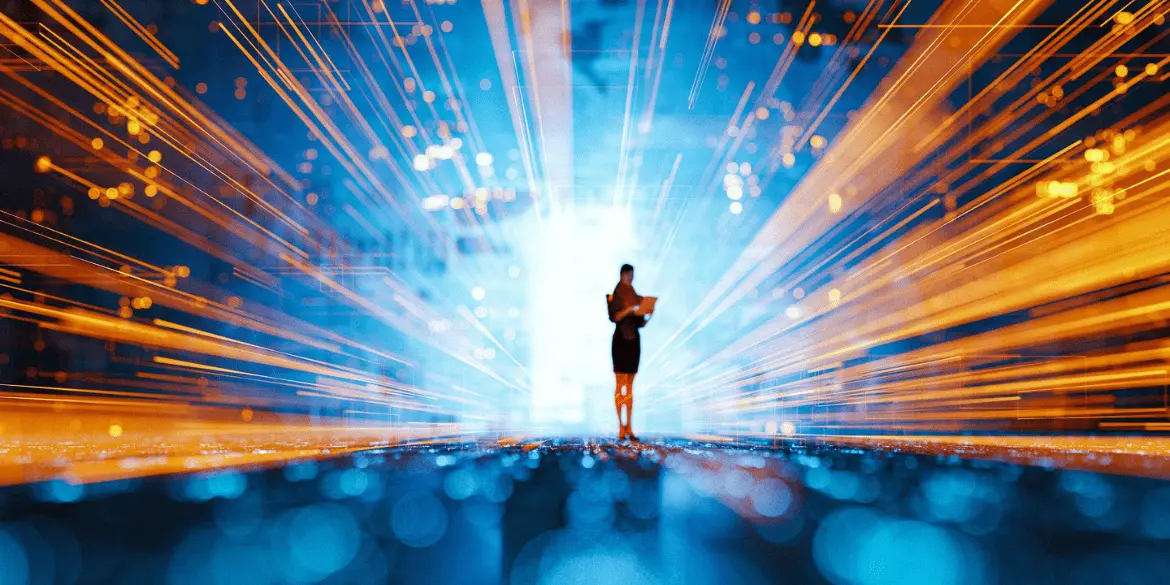
When AI applications go wrong, they can go very, very wrong. This is why we have an obligation to ensure that they are developed responsibly – that we understand the ethical implications of AI developments and have done everything possible to make them both effective and fair to individuals, communities and society as a whole.
To assist in this process, a variety of standards bodies are developing AI-related standards, including standards capturing principles and processes for responsible AI. In December 2023, ISO/IEC 42001 – the world’s first AI management system standard – was published, providing a framework of guidance for responsible AI development and use.
The principles of responsible AI
Even before this standard was published, many organizations that took the ethical implications of AI seriously had developed their own responsible AI principles. While they might use different terms for similar concepts or group them in different ways, the same themes cropped up again and again, demonstrating general high-level agreement about the principles that form the basis of responsible AI. These include:
- Transparency. Everything about the creation and implementation of AI, including how AI models make their decisions, should be clear and understandable by all stakeholders.
- Fairness and inclusivity. AI models should be designed to represent all user groups and treat all individuals fairly to avoid any form of bias or discrimination.
- Privacy and security. Those developing and using AI should respect and protect the rights of individuals to control their personal information, and should take the appropriate actions to safeguard sensitive data against any form of unauthorized access.
- Safety and reliability. AI models should function reliably, while ensuring the safety of all users and the wider environment. Any erroneous performance or malfunction should be detectable and rectifiable, with minimal disruption or harm.
- Accountability. Those developing AI must accept responsibility for how the AI models they create are used, and their models’ impact and consequences.
Applying responsible AI principles to AI training data
Since data is the foundation of AI development, responsible AI starts with the responsible preparation of AI training data. Here are some best practices:
- Transparency. Ensure that all your data methodologies are clear and understandable. You should be able to provide detailed information about the origin of the data, how it is collected, and the way it is used and processed.
- Fairness and inclusivity. Take active steps to combat bias arising from data issues. This includes scrutinizing data sources, preparing diverse and representative data (among other elements of data quality), and mitigating issues found during training by modifying the training data as necessary.
- Privacy and security. Always handle AI data in a manner that respects individual privacy. This includes complying with data privacy laws, obtaining informed consent where necessary, and implementing robust security measures such as encryption, access control and regular security audits to ensure the secure storage and movement of personally identifiable information (PII). You can also use techniques such as data anonymization or injecting noise into data to remove or obscure PII contained in datasets.
- Safety and reliability. Collect AI training data only from trusted sources and thoroughly verify and clean all the data before use, with measures in place to detect and address any inaccuracies or inconsistencies. You also need a way to add new data as relevant to keep pace with change. Other principles of responsible AI are also relevant here, since an AI model can only be reliable or protect the safety of users if, for example, it is free from bias and protected from a security breach that could compromise privacy.
- Accountability. Keep detailed records of data collection, processing and use, and implement rigorous auditing processes to monitor and evaluate data-related processes against the principles of responsible AI.
The bigger picture
Naturally, responsible AI principles apply well beyond the preparation of AI training data. They must be applied at every step of AI development, implementation, use and management, taking into account the ethical implications for all stakeholders – which is all of us. We need to take a holistic approach to AI to ensure that applications are developed responsibly and can make a positive contribution to society.
Connect with our TrainAI team to learn how we prepare AI training data responsibly to meet your unique AI needs.