Ensuring high-quality AI data: the foundation of AI success
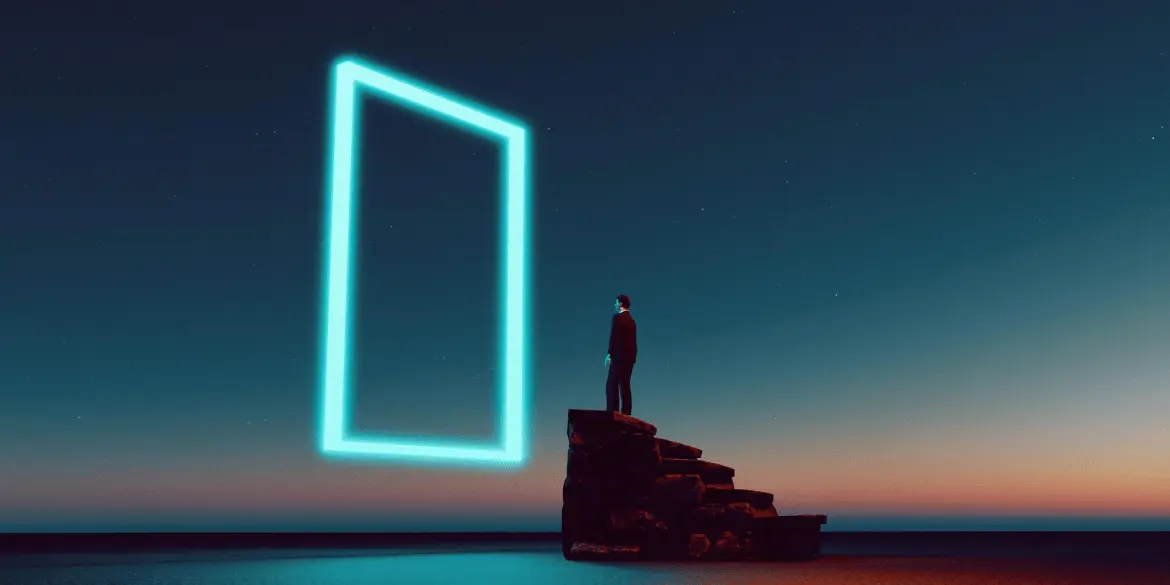
There's no shortage of stories of how AI can go wrong – with results that may be funny or serious, pleasing or horrifying. One thing these incidents often have in common is a problem with the data used to train the AI model. There are countless ways in which issues with AI training data – or the way it is prepared – can ultimately affect AI performance. This is why AI data quality is paramount: it can make or break your AI project.
So let's dig into AI data quality. What are the characteristics of high-quality training data? What is the real-world impact of poor-quality data, and the benefits of using high-quality data? And how do you ensure that the data for your next AI project is of sufficient quality?
What does high-quality AI data look like?
Through our work collecting, generating, preparing and validating data for AI training, we see directly how nuanced the issue of data quality is. High-quality AI training data should be:
- Accurate. As obvious as it seems that AI data should be up-to-date and error-free, it’s not so easy to be confident that this is the case. Badly labelled data is a common problem for AI projects, for example. Together with other data quality issues, inaccurate data can derail any AI initiative.
- Consistent. The data used to train the AI model should be consistent in format, structure and quality, because inconsistencies can cause the AI model to pick up on differences that shouldn’t make a difference – and learn the wrong thing. Ensuring AI data consistency can be especially challenging because the datasets can be so large, but it's an absolute must.
- Relevant. If you’re training an AI model to identify dogs in images, supplying it with images of cats won’t be relevant to the purpose and could confuse the model. Similarly, for a voice recognition model learning to understand English, audio data in French would not be relevant. Remember that the same data (or features of data) might be relevant for one purpose and irrelevant for another.
- Balanced. AI training data should include an equal spread of positive and negative examples so that the model can learn from both. Well-balanced AI training data prevents the model from being biased towards a certain outcome, leading to better performance when faced with real-world data after training. Balance and bias in AI data are clearly related.
- Comprehensive. Besides being relevant, AI training data should cover a broad spectrum of situations that the AI model might encounter when it's applied in the real world. The less comprehensive an AI dataset is for the intended purpose, the more likely the model is to respond inappropriately. For example, if a large language model is asked a question that its training data doesn't adequately cover, it will still generate an answer, but this answer may not be aligned with the real world. The wider the variety of relevant scenarios you train a model with, the better its performance will be.
- Diverse and representative. When creating a comprehensive dataset, it's critical to ensure that the data fully represents the diversity of those who will ultimately use and be affected by the model. This starts with data acquisition: if you're only using American data or only have Americans generating data, don't be surprised if it doesn't adequately represent Norwegians. Neglecting diversity will usually lead to the AI model failing to adapt to novel situations or different user groups.
- Bias-free. As explained when discussing the challenge of bias, bias can be so insidious that it takes specific focus and effort to catch and address it in AI datasets. Failure to do so risks the AI model producing biased decisions and predictions. It may prove impossible to remove bias entirely, but it’s important to do everything possible to minimize it, including ensuring that your AI training data is consistent, balanced, comprehensive, diverse and representative.
The different dimensions of AI data quality can overlap or be closely related. The root cause of bias might be a lack of balance, diversity or even consistency. Or a lack of consistency might create a problem of relevance. Even so, they are distinct concepts, as the following examples show.
When data quality goes awry
Here are three classic examples of AI gone wrong, used to illustrate the different dimensions of AI data quality.
Flawed facial recognition
An AI-powered facial recognition system fails to recognize dark-skinned people because it has been trained on a dataset comprising images of light-skinned individuals only. This limits its applicability and accuracy in real-world scenarios. Clearly the training data here is not:
- Diverse and representative, since the skin tones used for training are too narrow a spectrum for the intended use of the system.
- Bias-free, because of its lack of representative diversity.
Ruthless recruitment
An AI-powered recruitment tool, designed to choose the top candidates from job applications in a fair and unbiased manner, is discovered to be ignoring quality female candidates. The data used to train the model was a decade's worth of recruitment data that reflected historical gender biases in recruitment, where male candidates were favoured over equally qualified female candidates. Here the training data is not:
- Relevant to the system's purpose of equal opportunity employment, despite relevance in terms of job roles and industries. Because the data overwhelmingly reflected historically unequal employment opportunities, it was an unsuitable training set.
- Balanced, because it doesn't include a balance of examples of equally qualified women and men being selected.
- Bias-free, because of the historical gender bias reflected in the data.
Lousy loan practices
An AI-powered loans approval system is found to be denying loans to people from a specific location, even when they have a good credit history. Its training data is discovered to include a large number of past defaulters from that location. The AI has therefore learned to associate the location with poor creditworthiness, to the extent that this is overriding actual evidence of creditworthiness. Here the training data is not:
- Relevant – or at least, not entirely relevant. Geographic location has been inadvertently linked to creditworthiness, when it isn't a reliable signifier in individual cases.
- Balanced, because it lacks examples of creditworthy individuals from this specific location.
- Bias-free, because the issue of relevance has created a bias.
Benefits of getting it right from the start
Because high-quality data is the foundation of any successful AI project, it’s well worth investing time and resources to get it right. For those who develop, use and are affected by the AI model, the benefits include:
- More efficient development. Using high-quality AI data from the start eliminates or reduces the need to revisit data preparation to solve issues that occur during training. The time taken up front to ensure data quality is more than made up for by faster development and deployment of a reliable AI solution.
- Improved performance and decision-making. AI models trained with high-quality data are more likely to make good predictions or uncover genuine insights. Whether in a business setting, a hospital looking for a diagnosis, a predictive policing scenario or a household planning a holiday, more reliable AI performance leads to better choices made by the people acting on the model's output, and better outcomes for those affected by their decisions.
- Greater trust. More reliable and fair AI models naturally increase trust in AI, which is critical to the widespread adoption of AI technologies.
- Cost savings. Curating and cleaning AI data to ensure its quality might initially seem costly, but it can lead to significant savings in the long run, starting with more efficient development (faster, therefore more cost-effective). By developing a more reliable model you also reduce the risk of costly corrections of errors after deployment, as well as the costs of regaining customer trust and repairing the reputation of your brand. Fundamentally, high-quality AI training data saves money by preventing expensive mistakes.
How to ensure high-quality AI data
So what do you actually do to ensure that your AI project is using high-quality training data? Here are some best practices:
- Data governance. Establish a framework that outlines the responsibilities, standards and processes for data acquisition, preparation and management that will help maintain data quality.
- Data verification and cleansing. Implement a data verification process such as cross-checking against other sources, or using algorithms designed to identify anomalies and errors. Correct or remove all errors and inconsistencies found, and any duplicates in the data.
- Feature selection. Carefully consider all data points or features in terms of the different dimensions of AI data quality. For example, if building a model to predict housing prices, relevance would be served by including house-specific features such as number of bedrooms, location and square footage. Location-specific demographic data such as average household income or level of employment might also be relevant, but data about race or gender is unlikely to be relevant and may introduce a bias.
- Data audits. Conduct routine audits of the data to identify and address any quality issues. This should include bias auditing and mitigation, as covered in our blog on seven common AI training challenges.
- Continuous improvement. Keep the AI model up to date with new data that accurately reflects real-world scenarios, either as part of a continuous learning approach or through regular retraining.
- External help. Consider using third-party datasets that have been developed for specific AI models, or use an AI data service such as TrainAI from RWS to prepare high-quality AI data tailored to your unique requirements.