Issue #8 - Is Neural MT on par with human translation?

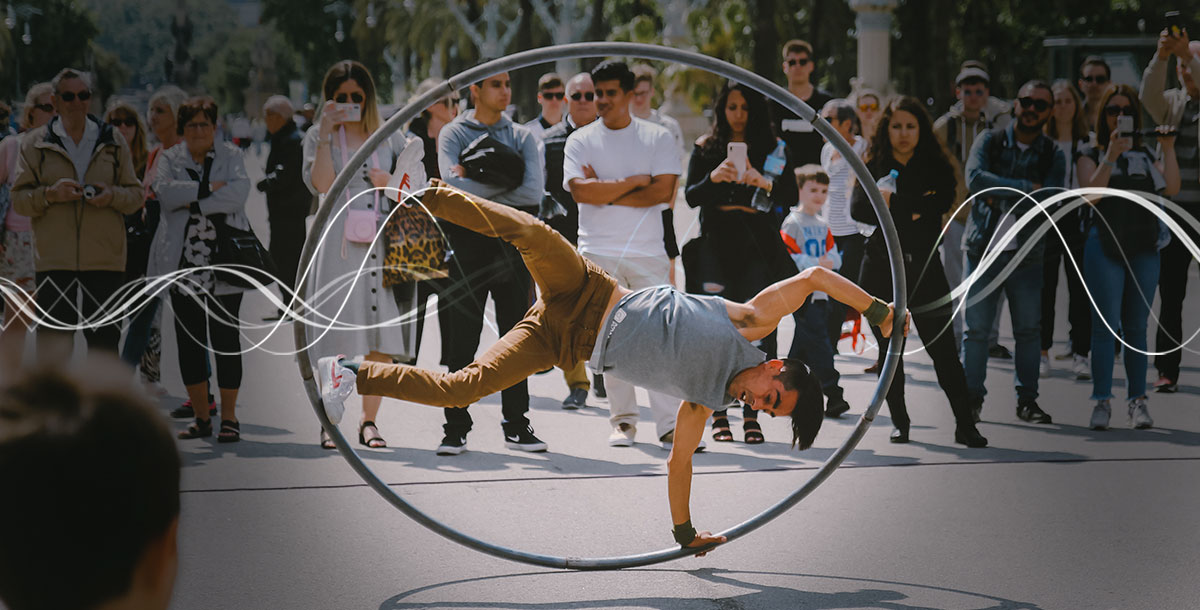
Introduction
The next few articles of the Neural MT Weekly will deal with the topic of quality and evaluation of machine translation. Since the advent of Neural MT, developments have moved fast, and we have seen quality expectation levels rise, in line with a number of striking proclamations about performance. Early claims of “bridging the gap between human and machine translation” stoked a lot of discussion in the translation community, but the recent bold claim of “achieving human parity” by Hassan et al. (2018) raised a lot of eyebrows.
This resulted in two teams of leading MT academic researchers taking a closer look at the latter claim, with some interesting findings. Let’s see what they discovered…
“Attaining the unattainable?”
In their paper, “Attaining the Unattainable? Reassessing Claims of Human Parity in Neural Machine Translation”, Toral et al. (2018) challenged three aspects of the evaluation that were not taken into account by Hassan, et al. (2018), namely:
- The language of the source side of the test documents;
- The proficiency of the evaluators;
- The availability of context when assessing individual sentences.
In discussing the first issue, Dr. Sheila Castilho, one of the authors of the paper, explained to us, “In their test, they were translating from Chinese into English. We believed that the quality of the data set could be playing a role here since 50% of it was originally written in English, translated into Chinese, and then back-translated into English. Therefore, the sentences originally written in English would be easier to machine translate than those originally written in Chinese since translated sentences tend to be simpler than their original counterparts.”
They found that this was indeed an influencing factor, with the machine translation output typically ranked significantly higher when the original input had been back-translated.
Assessing the Assessors
Regarding the proficiency of the evaluators, Dr. Castilho added, “We thought this could also be playing a role here since we have found in previous work that crowd-workers, as used by Hassan et al. (2018), tend to be more tolerant to MT errors than professional translators. What we found was that there was a big difference between the assessments performed by professionals compared to the ones performed by non-experts, where assessments by the professionals were more thorough and so they were able to find the widest gap between good human translations and the Neural MT."
“A Case for Document-level Evaluation”
The final issue relates to the fact that evaluations of translated output are typically carried out on a sentence by sentence basis. This point was focused on exclusively by Laubli et al. (2018) who suggest that, as MT continues to improve, translation errors become difficult to detect on the sentence-level, and that this can have a big impact when discriminating between human and machine output.
Replicating the assessments of Hassan et al. (2018), contrasting the evaluation of single sentences with entire documents, they found that “human raters assessing adequacy and fluency show a stronger preference for human over machine translation when evaluating documents as compared to isolated sentences.” This was also corroborated in the findings of Toral et al. (2018).
In summary
Laubli et al. (2018) suggest their results “point to a failure of current best practices in machine translation evaluation” and make the case for a switch to assessments on a document level. Toral et al. (2018) agree, and Dr. Castilho further asks, “How much can we trust our results if quality of our data sets are very poor?”
It is clearly beyond doubt that Neural MT continues to raise the bar in terms of quality. However, claims of human-like quality can serve to raise expectations of users beyond what can be achieved, and should be backed up with more robust evaluations.