Issue #38 - Incremental Interlingua-based Neural Machine Translation
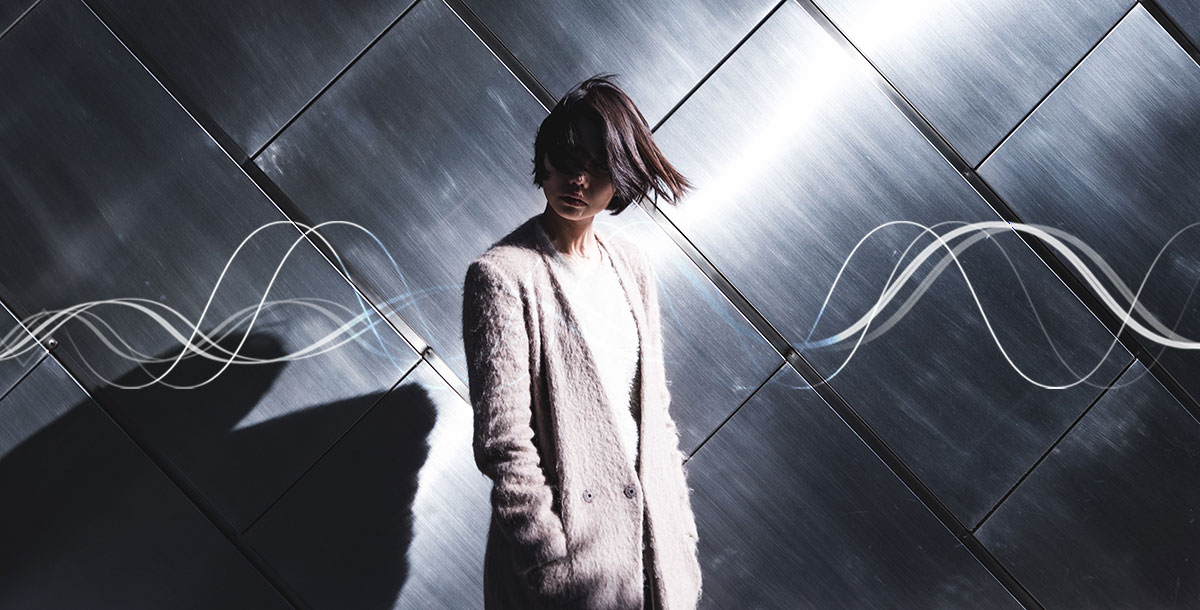
Introduction
Multilingual Neural Machine Translation is a standard practice nowadays. A typical architecture includes one universal encoder and decoder that are fed with multiple languages in training which allows for zero-shot translation in inference. The decoder is told which language to translate by simply recognising a tag in the source sentence that has this information. An alternative to this architecture is the use of multiple encoders and decoders for each language and sharing an attention layer which becomes the interlingua component. In both cases, components are trained at the same time and adding a new language implies to retrain the entire system.Joint training and Incremental Language Addition
We propose an architecture that allows to incrementally add new languages, refraining from training languages already in the system. For this, we propose an architecture of independent encoders and decoders and having one encoder and one decoder for each language. These encoders and decoders share the same intermediate representation.Let's assume we initially train our system with two languages (X and Y). To train a multilingual system with these two languages, we combine the tasks of auto-encoding in both languages (XX and YY) and translation from X to Y (XY) and from Y to X (YX). This is performed by optimising the auto-encoder losses from both languages (lossXX, lossYY) and the two translation losses (lossXY, lossYX). In addition, we compute yet another loss which minimises the distance between the intermediate representation of encoder X and encoder Y. We refer to this loss term as the interlingua loss (lossI).
Given the jointly trained model, the next step is to train a language Z without retraining any of the languages in the system. Having parallel data from language Z to either X or Y (let’s assume having parallel data Z-X, for illustration), we train a new bilingual system. We use the previously trained decoder X and train our encoder Z. Note that our decoder X is frozen and we only train the new module which is encoder Z. By doing this we are forcing encoder Z to produce similar representations to the already trained languages. As a consequence, our system is now able to translate from language Z to X and, in addition, we allow zero-shot translation between Z and Y because our architecture builds on compatible encoders and decoders.