Issue #22 - Mixture Models in Neural MT
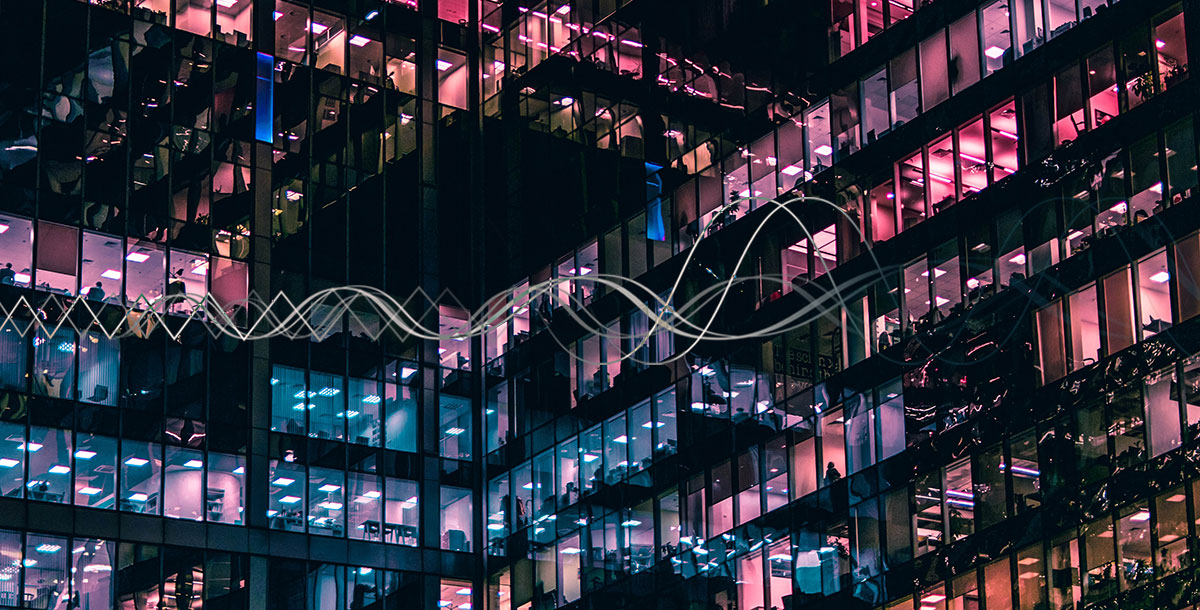
Introduction
It goes without saying that Neural Machine Translation has become state of the art in MT. However, one challenge we still face is developing a single general MT system which works well across a variety of different input types. As we know from long-standing research into domain adaptation, a system trained on patent data doesn’t perform well when translating software documentation or news articles, and vice versa, for example.
Why is this the case? Domain specific systems have lower vocabulary size, less ambiguities, reduced grammatical constructs and this lowers the chances of making mistakes. However, domain specific systems are inherently narrow in their applicability, and not suitable for a broader set of needs, as can often be the case in practice. In contrast to a domain specific system, a general (or generic) system is equally good at translating several domains but may not give the best translations on any one domain.
Can we combine the benefits of a domain specific system but train a generic system? Can we divide our corpora in several clusters and train many models and weight our models depending on the input? As we have many models, can we make them complementary? Let's take a look.
RNN Mixture Model
He et al. (2018) presented a so-called 'mixture model' based approach which tries to incorporate some of these aspects and can be seen as a serious attempt in this direction of research. They changed the simple neural MT architecture to incorporate diversity in the model. The approach is to have a neural MT system consisting of a set of translation models. During the training and the decoding time the system weights each model contribution. To keep the model simple, each model contributes equally, instead of depending on the input.
The mixture model is based on LSTM (RNN). The update of each LSTM unit depends on the previous state, the current input and additionally on a cluster vector. This additional cluster vector takes care of the mixture model implementation and is added in the decoding layer. Similarly, the softmax layer is also modified to take into account the cluster vector. The encoder part of the system remains the same and is shared by each model. Therefore, only single encoding is needed to get translations from all the models. As we can see, apart from the cluster vectors, the system shares most of the parameters among models.