Will AI translation technology replace translators?
10 Oct 2023
7 mins

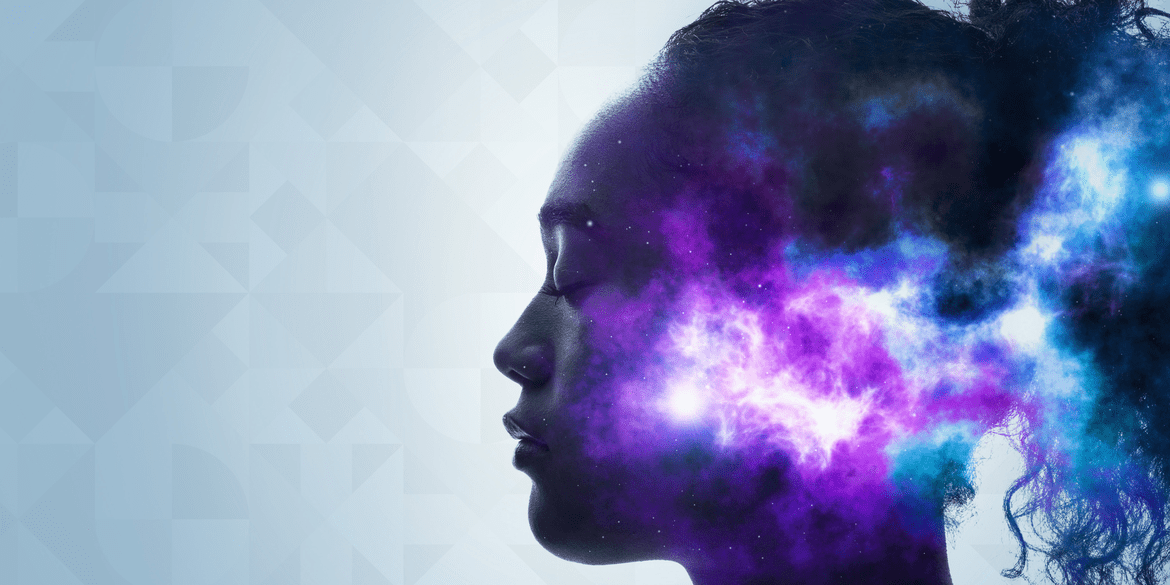
For as long as machine translation (MT) has existed (its origins stretch back to the 1950s, long before the advent of AI-powered translation technology), fears about the imminent demise of the human translator have been constant. And unwarranted. In fact, right now there is arguably a shortage of translators within the professional translation industry (see, for example, our latest Translation Technology Insights report).
But given the rise and rapid evolution of neural MT (NMT), and the even more recent arrival of large language models (LLMs) such as OpenAI's GPT, Google's PaLM (used in Bard), Meta's LLaMa and others, it's fair to ask whether the outlook has changed. Are the prophecies of doom for human translation now justified?
The short answer? No. We believe that fears about the power of AI to replace translators are still misplaced.
The longer, more nuanced answer? There's no doubt that the state of play is changing fast, and it would be hubris to declare that the role of translator will exist forever. But right now, looking forward with what we know about AI in translation, there are several reasons for translators to be optimistic.
AI-powered translation is both too imaginative and not imaginative enough
LLMs famously make errors known as hallucinations – and these are inherent in the way they work as generative AI models. In other words, they make stuff up – and there's no fix for this in sight. NMT doesn't suffer from hallucinations in the same way, but it does make its own types of errors. No AI translation technology actually understands any of the content it's translating, so embarrassing mistakes are inevitable. AI will translate metaphorical language literally, or entirely miss the point of humour, for example. The more comprehensive its training for a particular translation purpose, the less prone it will be to hallucination or error. But it's likely that there will always be contexts where accuracy matters too much to trust entirely to translation with AI alone.
Then there is the issue of bias – often caused by biases that exist in the data used to train both NMT models and LLMs. Again, this is an issue with no easy fix, not least because the biases that we're aware of are continually evolving. Not too long ago, systematic gender bias in language raised few eyebrows. We don't know what biases we have today that we're blissfully unaware of, that our children or grandchildren will be horrified by. Human input will always be needed to keep translation in line with evolving cultural realities.
Additionally, while we tend to think of bias as being (for example) about race, gender or ability, in the context of translation these are just particular types of a more general type of bias that can occur any time a word in the source language is ambiguous. This happens a lot, and can easily lead to failures in AI-powered translation. Lacking understanding, AI translation technology often just isn't as good as humans at detecting the intended meaning of a word from its context, Or the available context may just be insufficient to resolve the ambiguity, in which case only a human being can go and hunt up additional information to resolve it, or use their judgement to make the best call.
AI translation tech doesn't speak enough languages (yet)
English dominates the internet – and only seven or eight other languages can claim more than a 2% share of its content. But increasingly we see that businesses taking customer-centricity seriously want to translate into dozens of languages, up to 100 in some cases. For many if not most of these languages, AI powered translation can quickly reach its limits. This is because low-resource languages, especially in Africa and parts of Asia, don't have enough data available to train the AI models to recognize what good translation looks like. Without sufficient training, the quality of translation performed by AI can't reach a high enough standard for most contexts. It requires review and editing by a human professional.
There are workarounds to help improve the trainability of AI in low-resource situations, and it's certainly a focus of research. So this limitation may recede pretty quickly. Or it may prove to be more persistent than we think.
For now, the input of translators specializing in low-resource languages remains critical.
Audiovisual content is still tricky
Businesses are turning to animations and video – or other audiovisual formats such as virtual or augmented reality (AR/VR) – for a variety of reasons, including improving engagement on social media platforms, providing more effective customer support, and enhancing learning and development.
Translating audiovisual content is much less straightforward than translating text-only formats. First, consider on-screen text (OST). This often comes with space limitations that affect which translations will work and which won't. And if an OST word has more than one meaning, the context that can help disambiguate it isn't provided by other words, but by visual elements. These constraints make it tough for AI to do reliably well at translating OST.
Next, consider subtitles or closed captions. Even assuming that there's enough context here for reliable AI-powered translation, timing presents an additional layer of complexity. The captions need to match the visuals and, where relevant, the audio (translated voiceover or dubbed speaking roles, or for closed captions the sound effects). This doesn't just happen by magic and is notoriously difficult to automate. There has been (and will continue to be) some amazing progress in applying AI to subtitling, but it remains tricky – especially with the added complexity of translation. Whether or not you’re willing to rely on AI entirely will depend on your use case.
Translation with AI has a trust problem
People can be surprisingly accurate at recognizing AI-generated content – and because of its frequent errors, many are increasingly uncomfortable about trusting it. Especially in contexts where trust is critical, such as healthcare or finance, humans will be needed to make AI 'feel like home', not just in terms of accuracy but in offering a style and tone that is appropriate to the target audience, context and culture.
Remember, too, that translation with AI can only draw on content created in the past, used to train the AI models. But human knowledge and language are always evolving, and if AI training doesn't keep pace, AI-generated content will inevitably start to feel less and less human and relevant. So where does new training data for AI translation technology come from, if not from us? As one of our clients has said, "nothing we do stands still, and we need humans to tell the machines how we’re evolving our products, language and style.” As long as humans keep innovating, we believe human translators will remain central to breaking down language barriers.
Looking for a localization service that knows how to balance the strengths of AI and human translation? Think global, speak local with RWS Language Services.