RWS at AI Summit NYC: Building trust in AI through data quality

The AI Summit in New York was a whirlwind of innovation, insights, and thought-provoking discussions about the future of artificial intelligence across a range of industries and applications.
RWS was proud to be a part of this collaborative event, sharing our expertise and connecting with fellow AI enthusiasts. For those who couldn't make it or those who want a recap of the part we played, we've got you covered. Here's a look at RWS's key contributions and the major trends that emerged from the AI Summit NY.
RWS takes the stage
- Chris Doris, Executive Director - Product Management and Architecture - Morgan Stanley
- Ravi Narang, Head of AI/ML Engineering - Vanguard
- Sharad Varshney, CEO and Founder - OvalEdge
- Dmitri Sedov, Group Head of Product, Data & Analytics - LSEG
- Jorick Van der Hoeven, Head of Analytics (Finance) - Revolut
- Vasagi Kothandapani, President, Enterprise Services and TrainAI - TrainAI by RWS
- Vidhya Sekhar, EY Americas Financial Services Data and Analytics Leader - EY
Vasagi, alongside these other experts in the field, shared valuable insights on topics such as data governance, bias detection, and the importance of human oversight in AI. Her contributions to the discussion provided valuable insights into the importance of data quality, ethical considerations, and responsible AI implementation.
Let's dive deeper into the key takeaways from this insightful session.
Why data quality is foundational for AI in financial services
After setting the stage for a dynamic discussion by emphasizing the critical role of data quality in building trustworthy AI systems, Vasagi highlighted the unique challenges faced by the financial services industry, where accuracy, ethics, and compliance are paramount.
"In an industry like finance, which is a highly regulated industry, we are talking about ethics and compliance. We are talking about building fair AI systems," Vasagi explained. "The pillar of building these systems is the quality of the data."
Essentially, data is quality. So, data is the focus. To trust ML models to avoid hallucinations and give stakeholders reliable outputs with full context, the backbone is the data – the quality itself.
The panel discussed various aspects of data quality, including:
- Data governance: The importance of establishing clear policies and procedures for managing data throughout its lifecycle.
- Bias detection: The need to identify and mitigate biases in data to ensure fair and unbiased AI systems.
- Data accessibility: The challenge of accessing and integrating data from various sources and systems.
- Metadata management: The critical role of metadata in understanding and interpreting data.
The panelists emphasized the importance of a collaborative approach to data governance, involving data scientists, business owners, and legal teams to ensure that AI systems are developed and deployed responsibly.
The importance of explainability and transparency
A key theme that emerged from the discussion was the need for explainability and transparency in AI models.
The panelists stressed the importance of being able to understand how AI models arrive at their decisions, particularly in industries where trust and accountability are crucial. They also highlighted the need for clear documentation and communication around data lineage and provenance to ensure transparency and traceability.
This emphasis on transparency extends to the data used to train and fine-tune AI models, as understanding the origin, quality, and potential baises of training data is essential for building reliable and ethical AI systems. By providing clear documentation and insights into the data used in AI development, organizations can foster trust and accountability in their AI applications.
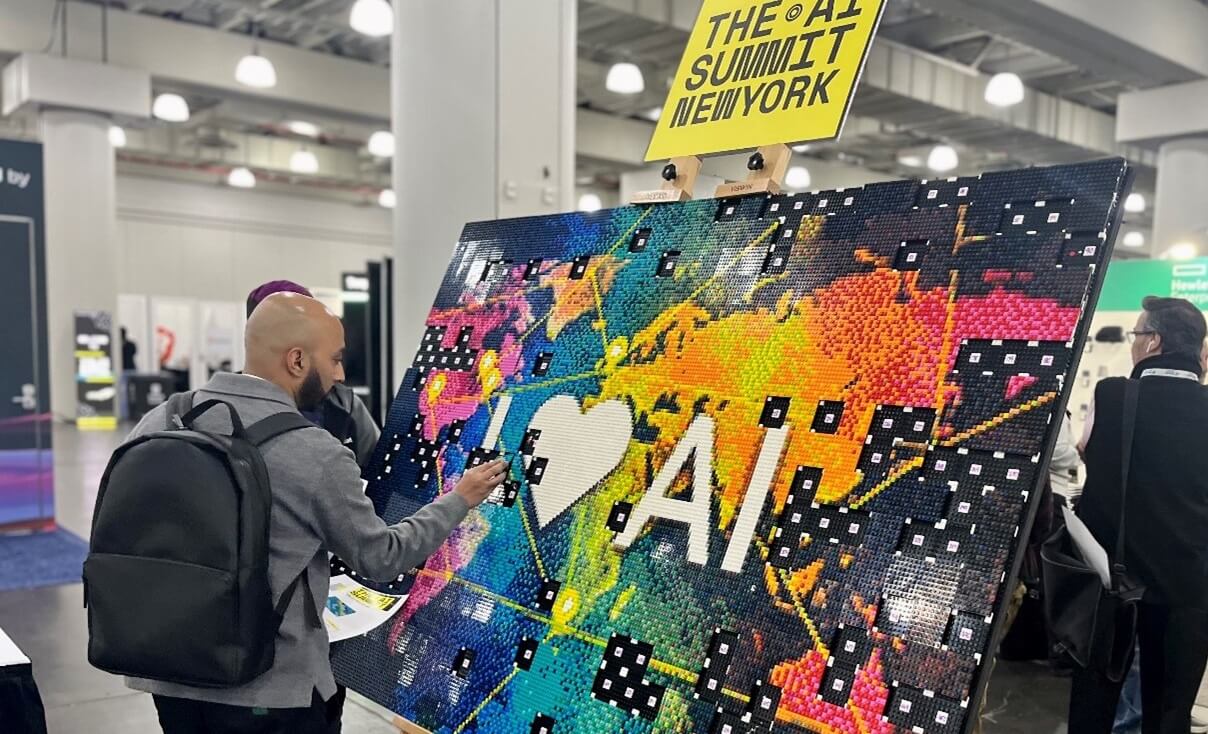
AI's role in enhancing data quality
The discussion also explored how AI can be used to enhance data quality itself. The panelists discussed various techniques, such as data labeling, statistical analysis, and feature engineering, that can be used to improve the accuracy, consistency, and completeness of data used for AI applications.
"AI can help in pre-processing the data," Ravi Narang, Vanguard noted. "You will start, first of all, with cleaning the data, normalizing the data, harmonizing the data so that it can integrate well."
This highlighted the potential for AI to not only analyze data but also to actively improve its quality, leading to more reliable and trustworthy AI systems.
Beyond finance: The wider implications for AI and data quality
While the session focused on the financial services industry, the key takeaways and principles discussed are applicable across a wide range of sectors. Any organization looking to implement AI, regardless of industry, can benefit from prioritizing data quality, governance, and transparency to ensure responsible and successful AI adoption.
The need for explainability and transparency in AI models, for example, is not limited to finance. In any sector where AI is used to make decisions that impact people's lives, it's crucial to be able to understand how those decisions are made. This is especially important in areas like healthcare, education, and social services, where AI systems can have a profound impact on individuals and communities.
Similarly, the importance of data quality extends far beyond finance. Accurate, complete, and unbiased data is essential for building reliable and trustworthy AI systems in any domain. Whether it's an AI-powered healthcare diagnostic tool, a self-driving car, or a customer service chatbot, the quality of the data used to train and operate these systems will directly impact their performance and effectiveness.
Partner with RWS for responsible AI
Want to learn more about how RWS can help you navigate the complexities of AI and data quality? Contact our TrainAI data solutions experts today to discuss your specific AI data and GenAI fine-tuning needs and explore our range of AI data solutions, including our data collection, annotation and validation, and GenAI fine-tuning services.