Beyond linearity: A new approach to measuring translation quality
29 Sep 2023
4 mins
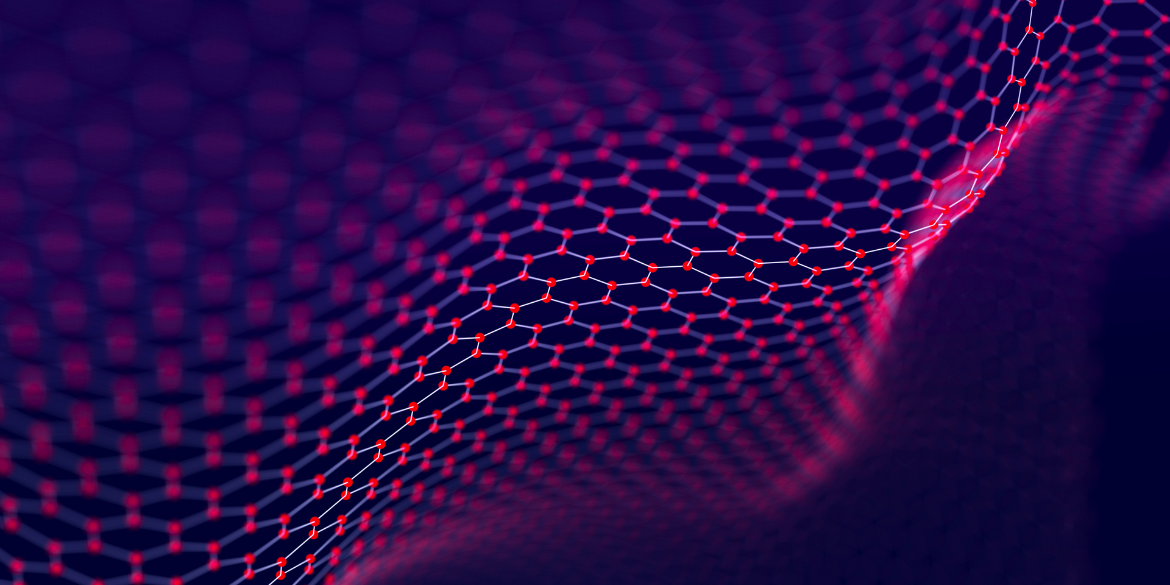
The industry-standard metric for measuring translation quality is linear in that it disregards the size of the reviewed sample. A single error found in a 100-word sample is just as bad as 10 errors in a 1000-word sample. Here we share some research and insights from RWS’s Innovation Lab, who investigated the dependency between error counts, sample sizes and the overall quality perception in LQE reviews. The result is surprising and reveals that reviewers become more sensitive to translation errors as the reviewed sample size increases, thus suggesting a non-linear dependency.
The linearity of the MQM 2.0 quality metrics
In the world of Linguistic Quality Evaluation (LQE), the Multidimensional Quality Metrics (MQM 2.0) has long been established as the industry-standard framework for measuring translation quality. At the base of this metric lie two key components:
- The total number of errors points found in the translation (called "Absolute Penalty Total")
- How many words there are in the translation being checked (called "Evaluation Word Count")
To obtain a quality score, MQM applies the following formula:
Overall Quality Score = (1 – (Absolute Penalty Total/ Evaluation Word Count)) * Maximum Score Value
Leaving the technical details aside, the MQM 2.0 formula is a linear function. No matter the size of the checked sample, the translation quality is evaluated in the same way. For example, a 100-word translation with one error is considered equally bad as a 1000-word translation with ten errors, which in turn is just as bad as a 10,000-word translation with hundred errors. The reason for this is that in all three cases, we have 1 error reported per 100 words. This linear approach is fundamental to MQM 2.0 but raises the question whether it accurately reflects how humans perceive linguistic quality.
Human perception of errors may not be linear
There are reasons to believe that it may not. The evidence comes from theoretical linguistics, specifically, from Charles Yang’s research on child language acquisition.
Yang's research suggests that children have a dynamically changing threshold for tolerating deviations from linguistic rules (i.e., “errors”). Yang labels this principle the Tolerance Principle. The most interesting property of the Tolerance Principle is that the threshold for tolerating deviations from language rules is not a linear function, but a log normal function of the sample size. Put simply, the larger the volume, the smaller error rate is accepted.
Should this principle be applicable to the way people experience linguistic quality, then 10 errors in a 1000-word translation sample would be perceived as worse quality than 1 error in a 100-word sample. This prompts the question: is a linear LQE metric the right one to use?
Unveiling the hidden pattern of quality perception
To find out the answer, the RWS research team analyzed 8,000 LQE reviews of varying sample sizes. The study aimed to establish whether the dependency between the recorded error count and the corresponding subjective quality experience changed in relation to the size of the evaluated translation sample.
The findings revealed a clear trend: as the sample size increases, reviewers become more sensitive to linguistic errors. For instance:
- In large samples (2-4K words), a quality experience score of 80 correlates with 8 normed penalty points.
- In small samples (less than 500 words), the very same experience score of 80 correlates with 15 normed penalty points.
Therefore when checking the quality of small translation samples, reviewers tolerate almost twice as many normalized errors compared to large samples.
The sample size matters
The study suggests that the Tolerance Principle is reflected in reviewers’ perception of language quality. The smaller a reviewed sample is, the better quality experience reviewers have for the same normalized error score.
The practical implications of these results include conducting LQE reviews on typical text lengths that users encounter. Additionally, a non-linear LQE metric can address the challenge of failing LQE score when the reviews are done on small samples – the nightmare of every Quality Manager.
To establish the ultimate non-linear LQE formula, however, there is certainly need for more extensive research. If you want to learn more, check out my full article in the September issue of MultiLingual magazine.