Neural machine translation vs large language models: Are you choosing the right AI tool for the job?
23 May 2024
4 mins

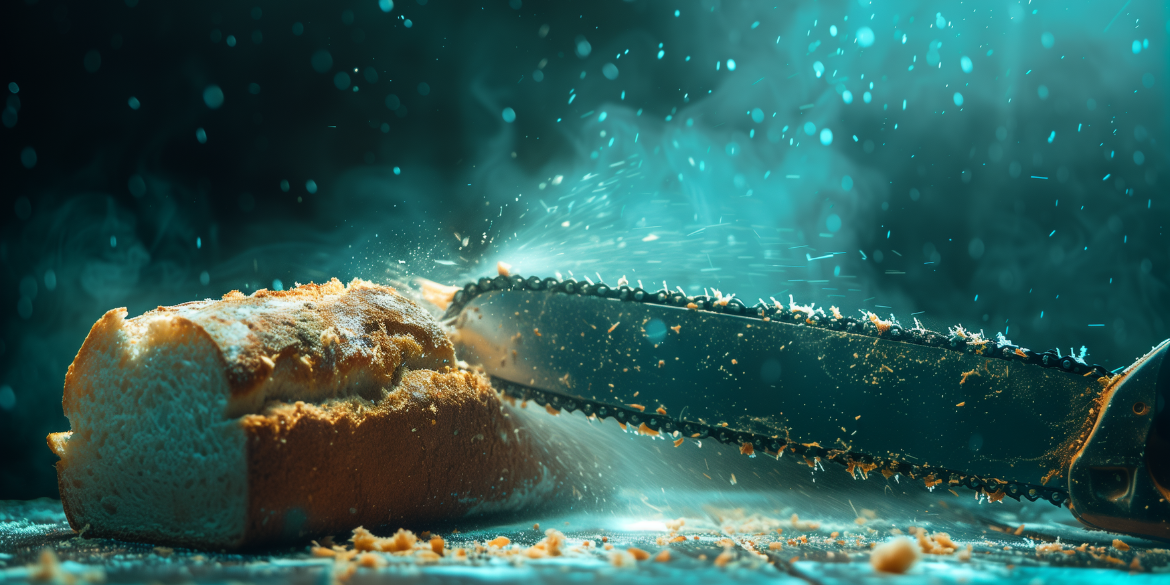
You wouldn’t use a chainsaw to slice a loaf of bread. And there’s no way you’d hop in a Formula One car for your daily commute – there are much more practical alternatives already available. So why invest in a brand-new, highly complex technology like a large language model to translate your content when neural machine translation is already offering you the power of AI?
For months, everyone’s been talking about generative AI and large language models (LLMs) and their potential impact on hundreds of different sectors (us included). In our world, this means a lot of people are asking whether AI can translate and localize.
The answer is yes – but that’s not a recent capability introduced by LLMs. AI has been a core component of translation technologies like neural machine translation (NMT) for years, enabling secure, accurate translation at scale for organizations around the world.
With the introduction of LLMs, we’ve got two forms of AI in play here, both with different strengths and capabilities. So which should you be using to translate and localize your content?
There’s a tool for every task
Despite the title, this isn’t really a case of pitting two technologies against each other. In fact, the same transformers used in LLMs were originally created for NMT. In reality there are situations where NMT is the right tool, and there are situations where an LLM could give you the best results. Whatever you’re trying to achieve, success is contingent on knowing which tool is best suited to the job and applying it in the right way.
Let’s break down a few common use cases to see where NMT and LLMs are most useful – and understand why.
- NMT: Translating in specific domains. For industries like technology, legal and life sciences, where accuracy is vital and a lot of the content uses domain-specific terminology, a specialized, adaptive NMT solution wins out. It’s possible to train and fine-tune a custom LLM to serve a niche – but that’s a high-effort approach that’s often cost-prohibitive.
- LLM: Highly nuanced source texts. Because LLMs are constantly learning from context and refining their own output, they’re starting to show real prowess in translating complex content that features cultural expressions and individual nuances. Without a human linguist involved, some MT solutions can struggle with the unusual metaphors and idiosyncratic phrases that make direct translation difficult.
- NMT: Translations in multiple languages. Many global organizations have key departments scattered across the world. For example, the legal team may be in the US, with European R&D labs and manufacturing facilities in China. With years of development behind them, NMT solutions offer broad language coverage (ours can translate more than 60, for example), while LLMs have mostly been trained using English resources so far. OpenAI’s GPT-3, for example, was trained on more than 90% English text.
- LLM: Large-scale translation requirements. For LLMs to be really effective, they need a lot of processing power behind them. But with the right resources, they can bulk translate enormous amounts of content – while considering context – in a matter of minutes. With Generative AI allowing users to create vast quantities of content rapidly, an LLM could be the best bet to keep up with the translation requirement.
- NMT: Secure, embedded translation capabilities. Through an API, organizations can embed NMT capabilities virtually anywhere in their business. Language Weaver clients, for example, are already using NMT to translate closed captions, live customer service interactions and product documentation. And because it’s an enterprise-grade solution, Language Weaver is highly secure – the most popular LLMs (such as OpenAI’s ChatGPT) are freely available but risk exposing business data.
- NMT and LLM: Improving the quality of translated output. We’re using our own LLM to add an automatic post-editing phase to our NMT output, combining the technologies for a more complete AI-powered translation process. Based on quality rules set out by expert human linguists, our LLM can automatically iterate on a translated text to improve accuracy where needed. This reduces the workload for reviewers and accelerates the whole workflow.
It's been AI all along – and it’s evolving all the time
We could go on for pages and pages exploring individual use cases for NMT and LLMs. But it all comes down to one simple matter: it’s all powered by AI at its heart and, like any technology, both are only effective with the right implementation.
The localization industry is still in the early stages of exploring how LLMs and generative AI capabilities fit into the translation puzzle. It’s so easy to get caught in the hype, but it’s vital that we focus on matching the tool to the requirement.
In most cases, NMT offers the combination of accuracy, coverage and power organizations require for their application. Some NMT solutions, such as our own Language Weaver, already incorporate the adaptive, context-aware capabilities and large-scale processing capacity that are tempting decision-makers towards LLMs.
If you’re interested in digging deeper into how the two technologies compare – their similarities, differences and combined potential – we’ll be discussing this topic more in our upcoming webinar, Beyond the Buzz: Understanding AI-driven Machine Translation.