How to future proof today’s machine translation
09 Jan 2023
7 mins
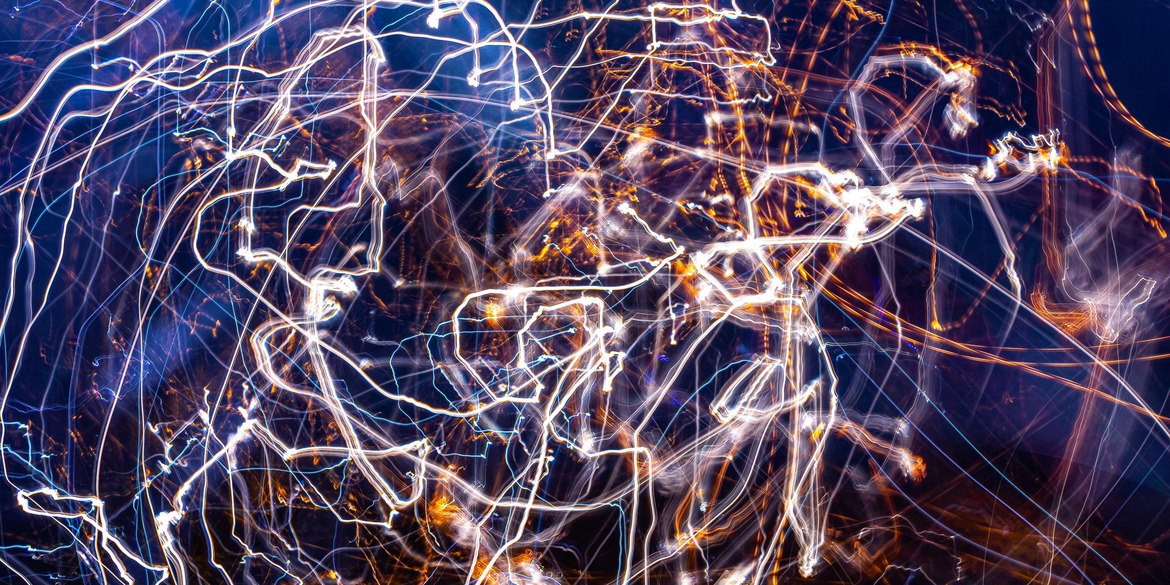
In 1980, the American writer and futurologist Alvin Toffler published a book entitled The Third Wave. The book talked about the transition from the industrial age (second wave) to the information age (third wave), describing some characteristics of the future society: a move from standardization to personalization, the gradual loss of societal consensus, and the eclipse of manufacturing goods by manufacturing knowledge. The world we live in now is not that far from Toffler’s predictions.
On the knowledge production front alone, it is estimated that each day our human civilization generates about 2.5 quintillion bytes of textual content. I have no way of verifying that estimate – nor do I remember where I read it. Quintillion is a word that sits towards that funny end of the scale where quantities are so huge that they are difficult to imagine. It is 10 to the power of 18: one million trillion or one billion billion. How many bytes is 10^18? More than there are stars in the observable universe. Or, to make it more tangible: if you printed one day’s worth of text on a stack of standard letter paper, your stack would be almost 2 miles high. That’s a lot of text (and a lot of paper).
It may be difficult to believe that we are such prolific communicators but, for illustration purposes, consider just a single tiny sliver of human activity: let’s say a car company is launching a new model. Throughout the whole process, there are hundreds of categories of content, each with hundreds or thousands of documents, that will be created, reviewed, published, revised, and published again and again – all to support the new product throughout its lifecycle. Research papers, design specifications, internal correspondence, shipping manifests, manufacturing plans, press releases, regulatory disclosures, test tables, brochures, flyers, websites, articles, price lists, advertisements, order forms, dealership instructions, training courses, finance information, sales reports, owner’s manuals, parts catalogs, service bulletins, contracts, warranties, disclosures, reviews, complaints, user forums, blog posts... And that’s just one product, from one company, in one industry. That two-mile paper stack does not seem such a stretch after all.
And yet, somehow, a portion of that content needs to, and will be, translated – on time and within budget, drawing on a population of professional translators currently estimated to be only about 500 thousand people strong. Even if we count translators not affiliated with any service providers, the number is going to be about 5 million people. That’s right – only about 0.0625% of the population of this planet is considered professionally involved in translating all the text the rest of us – including corporations, governments, and other entities – produce every day.
How is that possible? Well, we did not always generate that much content – that prolific production is one of the artifacts of the digital era, the information age. And, perhaps not surprisingly, as soon as the content explosion started, the translation industry responded with innovation, and transformed itself to meet the challenge with new technologies and new workflows. I think so far, we have witnessed three distinct waves, let’s call them tech revolutions, that changed the way professional translation work is conducted.
First Revolution – Translation Memory (TM)
Even though translation memory was invented in 1970s, our story begins in late 1990s when TM technology was maturing and quickly becoming a viable business solution. Its first adopters were language professionals: the translator community. Initially, it was mostly freelance translators and translation agencies, the “work doers”. Despite their initial limitations (expensive, clunky, and often buggy) the early TM systems could address the core problem of the human translation process: limited throughput, the stubborn ceiling of 2,000 words per day.
TMs automatically and reliably captured translations from individual contributors or small teams, and recycled them for subsequent, similar projects. For repetitive, iterative publications – like most tech publications – it was the way to go. For the language translation community, TM was a revelation, the killer app, a force multiplier that lifted the age-old human capacity limitation. It was the first translation revolution.
Second Revolution – Translation Management System (TMS)
The next phase was a direct consequence of translation memory proliferation. At some point it was only natural that the use of TM technology should extend to the content owners, the “work givers” - who themselves sought to directly reap the benefits of TM. Client organizations could use the same technology which isolated content that had not yet been translated and automatically translate the content that had, including even fuzzy matches that required only minor edits. And thus, TM travelled further upstream in the value chain, empowering the corporate customer. High tech, manufacturing, life sciences – any industry with global aspirations and a well-developed, iterative publication culture (user guides, online help, owners’ manuals, product descriptions) became relevant beneficiaries.
However, this new development had a set of interesting side effects. For starters, the small, local TM model was no longer adequate. To scale, one had to find efficient ways to work with large teams: for example, distribute assignments (bilingual pre-translated files, project translation memories, terminology databases) from work givers to work doers. Then, one had to collect the completed, or partially completed, bilingual work from various parties (translators, editors, reviewers) and make sure it could be efficiently reused. One had to merge multiple bilingual projects into a single multilingual deliverable. Parties had to agree on how to quantify the effort, which required standardization of the process, task, match, and rate definitions. One had to figure out how to notify project participants that there is work waiting for them. The translation world needed a centralized TM, a standardized workflow, and reliable automation. Out of that necessity a new category was born – a translation management system (TMS): the second translation revolution.
Enter Neural Machine Translation (Neural MT)
In the end, the second revolution consolidated the value of the LSP industry that now provided competitively priced, high quality human translation services to customers. For corporate customers, managing human value-added translation at scale remained a tricky proposition. Large localization programs, managed by service providers, are predicated on the assumption that the value produced by the translation process comes from human translators - because they are the only party capable of producing the sufficient output quality. These programs are complex and require expertise in maximizing the value of the human supply chain, and therefore are best outsourced. LSPs, after all, have become the longstanding experts in managing complex linguistic supply chains.
However, all that changed when Neural MT joined the game, triggering the third translation revolution. MT is now where TM was at the beginning of this story. It is making a difference, shaping the future of the industry. However, this time there is a crucial difference. Unlike TM or TMS, MT is not bound by the confines of the translation industry. The reasons for this are multi-faceted, having to do with increasing MT quality, changing customer expectations, and growing use cases where human translation is not practical because of volume, speed, and cost.
With neural machine translation, the real focus now shifts onto utility and usability. For many use cases and content types, MT quality (especially if the models can be adapted), is fit for purpose – if those models can be easily applied to the content that needs to be translated. That means getting closer to the source content, integrating with the native content platforms, supporting the relevant file formats, and providing new ways to incorporate human feedback. This has profoundly serious implications for the future.
If MT can (mostly) handle most of the content, then perhaps the whole paradigm needs to change. In the machine-first world, does it make sense to default to processes and platforms optimized for the human translation supply chain? Ultimately, translation itself is means to achieving an end goal – the end effect of translated text on the target audience: selling a product, promoting a brand, empowering the user to get technical support, etc.
The near future of translation is likely to be shaped by the trends we are observing already:
- A lot of content categories will be competently – if not perfectly – translated by NMT without human intervention, providing fast and inexpensive on-demand service.
- This may require something better than generic NMT models so NMT adaptation will play a bigger role.
- Knowing where NMT falls short and where human intervention is required will call for competent MT Quality Estimation.
- Translating non-public data will need models built with non-public data so the customers will want to run NMT adaptation on their end.
- That, in turn, will call for adaptation automation, since effort and cost of adapting custom models will need to be reduced.
- Big localization programs, even those with a substantial human involvement, will move to the NMT-first model.
- Professional linguists will contribute their expertise in new ways – overseeing and guiding machines rather than competing with them.
- As NMT becomes viable for more use cases, new interfaces for providing human feedback (other than post-editing) will be devised.
- The biggest customers will continue seeking professional linguistic expertise from their regular providers but the set of use cases will expand to new areas and require new skills and tools (e.g. content domain detection, content annotation, translation quality estimation, etc).
- At the same time, the audiences – the translation consumers – will continue showing preference for fast access to information vs. perfect translation quality. Speed will be key.
- As MT continues to provide utility, the determination of “good enough” translation quality will become a major business consideration – how do I know which content requires human intervention? How do I know how good the translation is? This, in its own right, may as well become the next frontier – and is perhaps worthy of a separate blog post.
- All of the content pressures (quintillions!) will only become more acute as Large Language Models and other technologies are used to generate quality content at scale.
If you agree with the points above – or at least with some of them – consider the following conclusions:
- As a content owner/producer/publisher/manager, you will be approached by an NMT provider with an offer to fulfill most of your translation needs by means of Neural MT.
- Understanding what NMT can and cannot do will be key – hence the importance of MT Quality Estimation and awareness of Human-in-the-Loop options.
- In the world of MT, one size does not fit all - so you’ll probably benefit from adaptive MT models that can keep up with your evolving needs.
- The NMT platform you select will need to be fast, scalable, and protect your confidential content.
- It will also need to integrate into your existing content infrastructure and provide capabilities beyond translation (language detection, summarization, entity recognition).
- All of those capabilities will have to be accessible via simple and intuitive UIs, predictable and well-documented APIs, with robust reporting – and come with a reasonable Total Cost of Ownership.