Data, content and language: The critical elements for successful AI projects
27 Nov 2024
6 mins

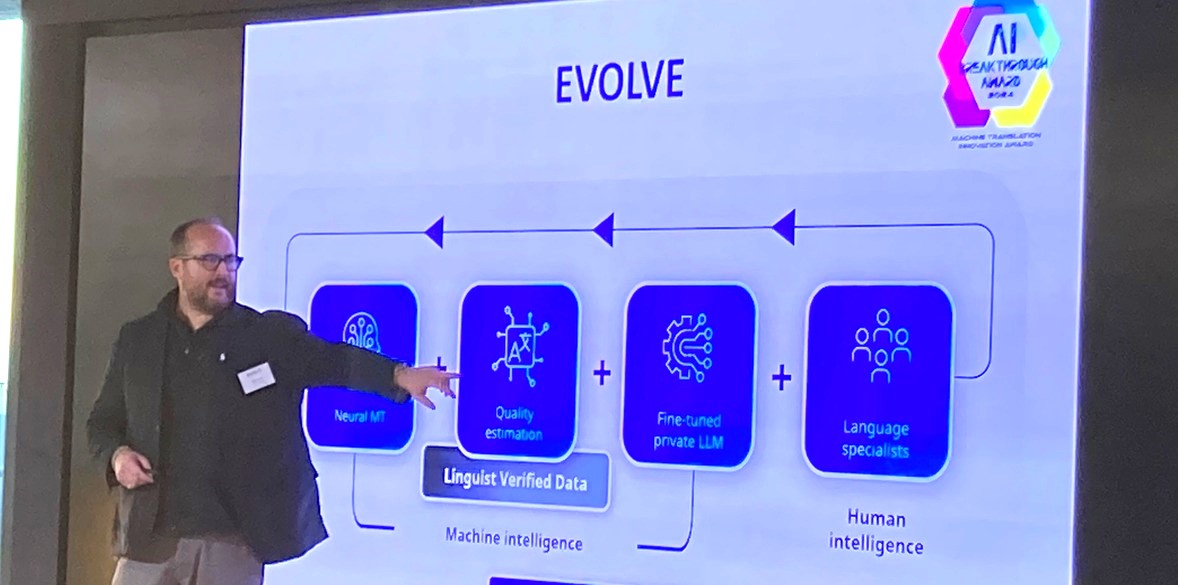
RWS recently hosted a sector-specific AI Showcase for Financial Services in London, held at the prestigious offices of leading asset management firm Capital Group. The event was designed to provide an overview of the key building blocks for delivering successful AI applications, some of which are frequently overlooked in the race for progress.
As pressure grows on firms to move from experimentation to operationalization, and more importantly from proof-of-concept to monetization, what are the critical success factors that drive this transition?
Subject matter experts from across RWS presented their three perspectives at the event: Data, Content and Language.
The data imperative
Lou Salmen from our TrainAI practice began by discussing how AI, particularly Generative AI (GenAI), is being utilized in financial organizations today. He highlighted use cases, challenges and the importance of multilingual data in AI development.
Some of the key use cases currently being implemented by finance firms were identified as follows:
- Fraud prevention: Using AI to analyze credit card transactions in real-time, reducing false declines.
- Process automation: Utilizing AI to analyze legal documents, significantly reducing manual work.
- Customer support: Employing chat-activated AI to handle hundreds of thousands of question variations for banking tasks.
- Forecasting and analysis: Utilizing GenAI and Large Language Models (LLMs) to assess the investment potential of companies as well as detecting blockers to them realizing that promise.
- Personalization and customization: using GenAI to personalize user experiences, including search results, product offers and loyalty programmes.
Achieving the transition from pilot to production is not without its challenges though, as any organizations currently engaged in this process would attest. Chief among these is:
- Language fluency: GenAI systems are often trained on data from a small number of major online languages, limiting their effectiveness in multilingual contexts.
- Production deployment: Only a small percentage of GenAI experiments are moved into production as shortcomings override potential, particularly for risk-averse companies.
- Impact measurement: Organizations struggle to define and measure the impact of GenAI initiatives, particularly in terms of return on investment (ROI)
- Data-related issues: Concerns about data privacy, security and the use of sensitive or confidential data slow down GenAI efforts.
So how can companies create a balance between the opportunities and challenges? Some key areas for attention were identified to support firms in developing this delicate equilibrium:
- Data importance: High-quality, targeted data is crucial for successful AI model development.
- Multilingual data: Leverage multilingual content and linguistic resources as valuable data for AI development. Localization teams possess valuable multilingual content that can enhance AI training so organizations should determine who owns language within their structure and involve them in AI training and evaluation.
You can find out more about how we are working with clients to achieve this balance by clicking here.
Content: The key to trust
Rachel Lindley from our Content Technologies group took over from Lou to emphasize the importance of structured content for trustable AI. She covered the challenges faced by clients, the role of retrieval-augmented generation (RAG) and the benefits of AI-driven knowledge portals.
The key challenges in the content lifecycle were identified as:
- Content creation: Authoring, localizing and managing content across multiple channels.
- Regulatory and ethical considerations: Ensuring content security and compliance, a particular challenge for finance institutions.
- Two-way content management: How to create a process for analyzing and responding to consumer-generated content.
In response to this, companies need to harness content for the specific needs of AI through the development of an AI-driven knowledge portal with the following characteristics:
- Content taxonomy, where structured categorization underpins RAG accuracy.
- Content governance, with standards and policies that ensure high-quality, up-to-date content.
- Metadata and workflow, with descriptive tags and organized workflows that enhance retrieval precision.
In achieving this, they can realize a host of practical benefits, including:
- Translation improvements: Lower costs through greater consistency and reusability.
- Delivery enhancements: Dynamic omnichannel delivery and personalized, contextualized results.
- Content development gains: Reduced costs, higher quality and better governance.
- Cost reductions: Content reuse, elimination of redundant content and lower risk through stronger governance.
Bringing order to content chaos across the organization contributes significantly to the transformative potential of AI in financial services by simplifying processes and supporting global growth. You can see an example of how this works in a financial services context by watching the short video here.
The explosive growth in linguistic AI
The third session, on language, was delivered by Matt Hardy from our Linguistic AI team. Matt explored the evolution of translation technologies and the integration of AI in language services, focusing specifically on the benefits of combining human and machine intelligence.
The current state is one of increasing demand for translated content but with a limited global pool of language specialists. Clearly the answer to this growing challenge is automation but different AI models are suited to distinct tasks. For instance, the latest neural machine translation (NMT) ticks the boxes for cost and speed but, for heavily-regulated content especially, some human post-editing intervention (preferably with domain expertise) is required to ensure the highest levels of accuracy. LLMs can now handle multiple linguistic tasks with better fluency but come with challenges like cost, inaccuracy and limited language support.
The answer lies in a combination of the best features of currently-available language technology and the expertise of trained human linguists. RWS has developed an industry-first, award-winning solution to achieve just that. It is called Evolve and it combines translation management, NMT, machine translation quality estimation (MTQE), a fine-tuned private LLM and language specialists into a single solution that offers the following benefits:
- Secure and strategic: Avoids risks associated with using public AI tools.
- Speed-to-market: Automates more of the translation workflow.
- Efficiency: Maximises efficiencies through the entire translation process, achieving gains of up to 65%.
- Quality at scale: Delivers consistent translation quality for large volumes.
- Self-improving: The system improves over time; the more you use it, the better it gets.
The video here introduces Evolve and how this streamlined model is transforming translation workflows for enterprise clients.
Data + content + language for foundational AI
The event brought clarity to some of the challenges faced by finance firms engaged in extensive AI initiatives, most notably that the success of their projects lies in ensuring that all of the component parts are in place before the transition can be made from pilot to production.
RWS possesses all of these parts – and we’re working with clients in multiple industries, including financial services, right now to solve their AI challenges. Find out more here or talk to us by filling in the form here.
You can also stay up-to-date with our most recent news for financial services via our LinkedIn page here.