The role of big data in drug discovery
04 May 2023
7 mins

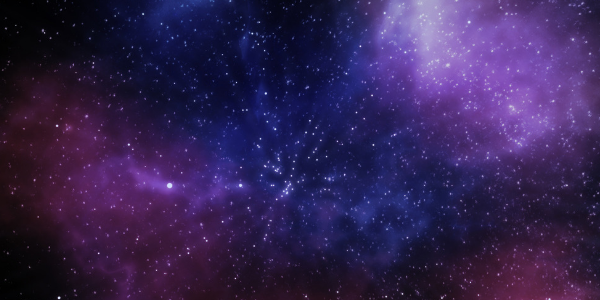
The pharmaceutical industry is constantly searching for new and innovative ways to improve the drug discovery process. One of the most promising approaches in recent years has been the use of big data. Drug discovery is an intricate and resource-intensive process that involves numerous stages, from target identification to clinical trials. Big data has the potential to transform every step of the drug discovery process by providing vast amounts of information and insights that were previously unavailable. A study estimated that the potential value of Big Data in the healthcare industry could be worth more than $300 billion per year in the United States alone.
Integrating Big Data into drug discovery enables scientists to analyze vast amounts of data, including genetic information, clinical trial data, and real-world evidence, to identify potential targets for drug development. This has the potential to streamline the drug discovery process, leading to the development of more effective and targeted therapies.
Big Data in preclinical drug discovery
Preclinical drug discovery is the stage where researchers identify potential targets for drug development. This stage involves a significant amount of trial and error as researchers test numerous compounds to identify those that show therapeutic potential. Big Data can potentially optimize this process by analyzing large amounts of biological and genetic data to identify potential drug targets and optimize existing ones.
One example of using Big Data in preclinical drug discovery is the Human Genome Project (HGP). The HGP is a major international effort to sequence and map the entire human genome. One of the biggest challenges of the HGP was dealing with the massive amounts of data generated by the sequencing process. Big data technologies were employed to store, manage, and analyze genomic data. This allowed the HGP to handle the enormous volumes of data and identify important genetic variations that contribute to disease. Additionally, machine learning algorithms were used to identify patterns in the data and predict the likelihood of certain genetic outcomes. The data generated by this project has enabled researchers to identify new drug targets and develop targeted therapies.
Big Data in clinical trials
Clinical trials are a critical stage in the drug development process. Clinical trials involve testing drugs in human subjects to assess their safety and efficacy. They generate large amounts of data, including patient demographics, medical histories, laboratory results, and treatment outcomes. By leveraging big data technologies, researchers can analyze this data to identify patterns and correlations that may not be apparent using traditional methods. Big Data can play a significant role in optimizing clinical trials, from patient recruitment to real-world evidence.
Big data analytics can help reduce the time and cost of clinical trials. By using machine learning algorithms to identify patient subgroups that are more likely to respond to a particular treatment, researchers can design smaller, more targeted trials that are more likely to succeed. This approach can potentially reduce the time it takes to bring a drug to market by up to 50%.
Real-world evidence (RWE) refers to data obtained from clinical trial designs, such as large simple trials, pragmatic clinical trials, and observational studies. It could include data collected from electronic health records and insurance claims. By analyzing RWE, researchers can design more effective clinical trial strategies that result in novel treatment options. Ultimately, this approach allows for the developing of new medical products that can significantly improve patient outcomes. It also helps speed up the drug development process and cuts down the overall costs.
Challenges of Big Data in drug discovery
While Big Data has the potential to revolutionize drug discovery, this approach also poses several challenges that need to be addressed. One of the most significant challenges is privacy. Privacy concerns arise due to the massive amounts of personal data that are generated and stored during the drug discovery process. Ensuring this data is handled ethically and appropriately to maintain confidentiality and privacy is a critical challenge. This is particularly difficult when data is collected from multiple sources, and data integration is necessary to produce meaningful insights.
Another challenge is bias. As with any data analysis, there is a risk of bias in the data being analyzed, leading to inaccurate or incomplete results. This can skew the data, leading to inaccurate conclusions and potentially harmful treatment decisions. Efforts to address these challenges include increasing diversity in data collection and utilization of machine learning algorithms that can detect and correct biases in the data.
Data integration can also be difficult as data comes in from a variety of sources, and so it can be challenging to integrate this data into a meaningful analysis. These challenges can lead to data accuracy, consistency, and comparability issues. Data integration requires significant resources, including specialized software and personnel.
These challenges require collaborative efforts from various stakeholders, including policy-makers, researchers, and the public. To overcome these challenges, appropriate policies, ethical standards, and guidelines must be established to ensure the responsible collection, integration, and utilization of Big Data in drug discovery. Furthermore, increased standardization, collaboration, and transparency are necessary to promote the accuracy, consistency, and comparability of data across various sources. Ultimately, addressing these challenges will enable the effective use of Big Data in drug discovery and lead to the development of novel treatments for various diseases.
Future prospects of Big Data in drug discovery
The true potential of big data in drug discovery lies in the field of precision medicine and personalized therapies. Precision medicine aims to provide targeted treatments tailored to individual patients' specific characteristics, such as their genetic makeup, lifestyle factors, and medical history. Big data can play a critical role in achieving this goal by providing vast information about patient populations and their unique characteristics.
By leveraging big data, researchers can gain a deeper understanding of diseases' underlying molecular mechanisms and develop drugs specifically designed to target these mechanisms. For example, by analyzing large-scale genomic data, researchers can identify specific genetic mutations that are associated with certain diseases and develop drugs that target these mutations.
One of the most exciting prospects of big data in drug discovery is the development of predictive models that can accurately predict a patient's response to a particular drug or treatment. By analyzing large-scale data sets that include patient demographics, medical history, genetic makeup, and other factors, researchers can develop algorithms that predict how a patient will respond to a particular treatment. This can help to identify patients who are most likely to benefit from a particular treatment and avoid exposing patients to drugs that are unlikely to be effective.
Another important aspect of precision medicine and personalized therapies is the ability to analyze data on patient populations which allows researchers to identify subgroups of patients who share similar characteristics and develop drugs that are specifically designed to target these subgroups. This approach has already been successful in treating certain types of cancer, where drugs have been developed that target specific genetic mutations that are only present in certain subgroups of patients.
As the field of big data in drug discovery continues to evolve, we can expect to see significant advances in developing novel drugs and treatments that will improve patient outcomes and lead to more effective and efficient drug development processes.
Click here if you would like to learn how to improve the efficiencies behind your clinical trials.