Tailoring AI-powered machine translation for your business
25 Apr 2024
4 mins
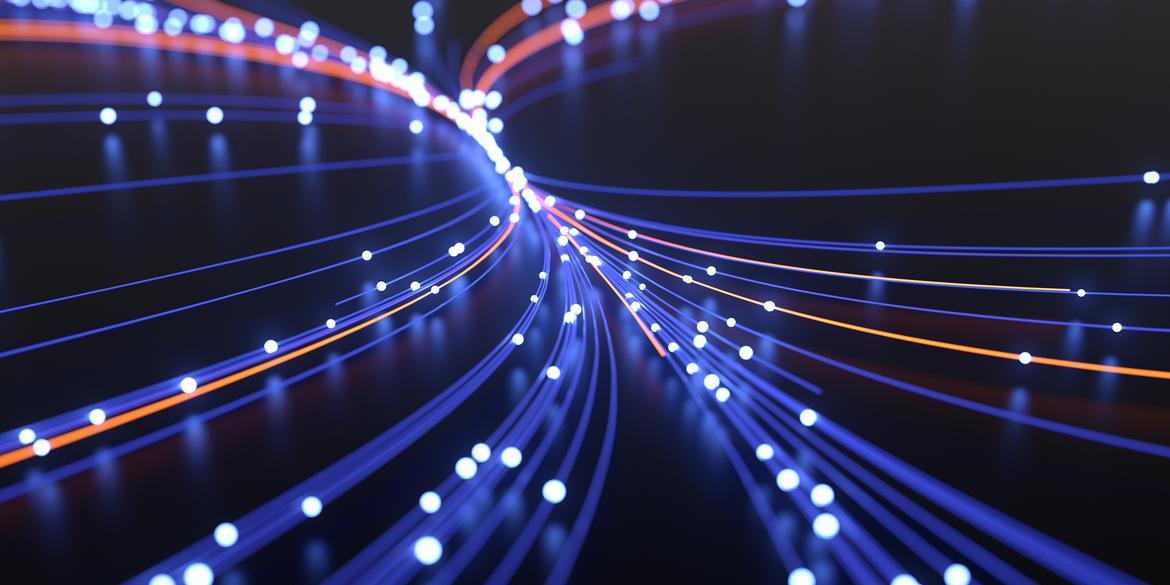
If you’ve ever hauled out your phone on holiday and used something like Google Translate to decode the local language, you’ve probably marvelled at how easy AI translation now makes it to navigate the world with understanding.
On the other hand, the (sometimes very funny) mistakes that you’ll typically encounter, while not hindering overall understanding, are a lesson in how complex human language really is. As incredible as AI translation has become, you don’t have to use it very long to appreciate how challenging it is to get reliably good translation from a machine.
Businesses need better
Faced with inconsistent machine translation quality while on holiday, we can just shrug (or laugh) it off. But that’s not an option for businesses communicating with audiences around the world in the diversity of languages that they speak. Customers expect to be treated with respect, and poorly or erratically translated content doesn’t feel like respect. Furthermore, the translation challenge is even greater for businesses, for two reasons:
- For many, the content they’re translating is peppered with industry terminology that is too niche for generic machine translation tools to handle well.
- Businesses have brand guidelines – specifying writing style and tone of voice, product names and descriptions, words preferred or to be avoided – that generic machine translation tools will simply ignore.
Does this mean that businesses must rely on human translation, despite the obvious cost and speed advantages of AI translation? Not at all – because generic machine translation is not the only option.
Instead, businesses are turning to adaptive machine translation (adaptive MT) and transforming their ability to produce MT outputs that are accurate, consistent and specific to their brand’s unique translation context.
What is adaptive MT?
Modern MT solutions use neural machine translation (NMT), an approach based on AI deep learning that allows the solution to improve its translation accuracy as it is exposed to more training data. The challenge for businesses is that the data used to train most NMT solutions is broad enough to produce what is essentially a multi-tool that is quite good at a lot of things, but not excellent at any. What every business really needs is an AI translation tool designed specifically for their translation context.
Enter adaptive MT, also known as custom MT, which allows users of a generic machine translation solution to modify the model they’re using with more specific data, so that the model’s output aligns more closely with their particular needs.
The ways in which you can adapt a model depend on the options offered by the developers of the generic machine translation solution that you’re starting with (more on this below). But the general idea is that over time the model learns the appropriate terminology and style specific to your industry and brand, morphing from a multi-tool into one finely honed to your translation context.
Different adaptive MT approaches
At a conceptual level, there are two ways to adapt an MT model:
- Overwrite and steer
- Fine-tune
Method 1: overwrite and steer
As the name suggests, when you overwrite and steer an MT model, you’re telling it to use information you provide to adapt its output – without affecting the underlying model at all. You’re steering it to deliver the output you prefer through explicit instructions that tell it to replace its output if it doesn’t match what you want.
Overwriting best-practice: fluent terminology
The classic way to do this, offered by most adaptive MT solutions, including Language Weaver from RWS, is through dictionaries or terminology lists. These let you specify the industry terminology or brand-specific language that you want to use (including names or terms that should NOT be translated). The adaptive MT solution knows that whatever output it generates through its default training, if the terminology you’ve given it specifies a different word or phrase, the terminology list must override its default output.
In Language Weaver, you can specify different dictionaries for different purposes and users can choose whether to apply an available dictionary or not. What we call ‘fluent terminology’ also ensures that the overwriting doesn’t depend on an absolute match. If, for example, your terminology list includes only singular nouns and present-tense verbs or verb roots, the solution will know how to use them in the plural or the past tense.
User feedback: good for overwriting, better for fine-tuning
Another way to overwrite and steer an MT model is through user feedback. Language Weaver, for example, allows users to edit the translation provided, and this feedback can be approved and captured as an additional overwriting resource for the model. But used in this way, it would only work through 100% complete matches going forward. To harness the power of user feedback properly, you really want to use it for fine-tuning.
Method 2: fine-tune
When you fine-tune an adaptive MT solution, you’re modifying the AI translation model itself by training it with new data targeted to your needs. Through this additional training, the model learns how to use information and language specific to your industry and brand (your translation context).
One way to do this is to have the provider of the solution develop a custom MT model for you, either using data you give them or by sourcing or creating relevant data for you. Language Weaver Custom and Expert options fall into this category.
Increasingly, though, businesses also want to be able to fine-tune their machine translation solution themselves. At Language Weaver we’re meeting this need for self-service adaptive MT through our
Adaptive and Auto-adaptive Language Pair options.
Existing translations or translation memories used for fine-tuning
Some businesses are lucky enough to have an existing corpus of approved translated content from known sources or, even better, existing translation memories (TMs) that store translations in an organized database. These can usually be used to fine-tune an adaptive MT solution, and at Language Weaver we make this even easier with an automated data cleaning capability. This helps you improve the quality of your TMs before you use them to fine-tune the machine translation model to align it with your translation context.
Terminology and feedback used for fine-tuning
If you don’t have any existing translated content or TMs, it doesn’t mean you can’t fine-tune an adaptive MT model – as long as it accepts other types of training data. For example, with Language Weaver you can use both terminology lists and user feedback as training data to fine-tune the model. With the auto-adaptive option this happens continuously, so every change to terminology or new piece of user feedback is immediately available to the AI translation model to learn from and improve its alignment with your specific translation context. Naturally you can set up workflows to ensure that only approved terminology and edits are fed to the model, and these can include auto-approval for feedback from trusted users such as professional translators (if you have them) or fluent subject matter experts.
The benefit here is that every person in your organization who provides valuable feedback is training your model for the good of the entire business. Because Language Weaver can translate entire documents and users can provide feedback on any part of the translated output directly within the Language Weaver interface, you can see improvements quite quickly, even starting from scratch with no pre-existing datasets for AI training. As long as you have active feedback loops in place, the more you use Language Weaver the better it gets at meeting your specific needs. Over time, the need for users to edit at all will reduce, because the solution’s default output will increasingly reflect your preferred usage.
How will you adapt?
Whether or not you’ve got existing translation resources such as TMs and terminology lists, the right adaptive MT solution can transform your ability to use AI translation to communicate across language barriers. No longer do you have to live with machine translation that doesn’t appreciate what makes your industry and brand unique. Instead, you’ll have a tool that will learn from you and adapt to you – and continue to do so as your business changes and grows.
For more on Language Weaver’s adaptive MT capabilities, watch our webinar: Enhancing translation outcomes with adaptive capabilities.