Realizing trustworthy AI through data
08 Mar 2024
4 minute read

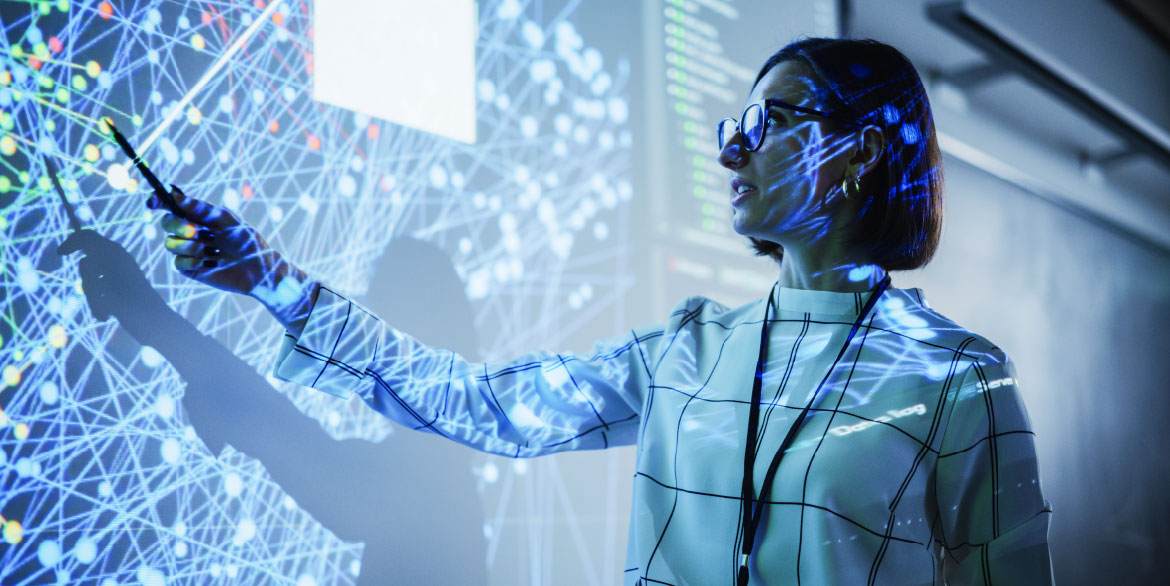
The use of artificial intelligence (AI) is rapidly increasing and its impact on our everyday lives can’t be ignored. However, with great power comes great responsibility – making it crucial to develop and use AI in a responsible, trustworthy manner.
Orders and regulations
Governments have recognized this need and have started implementing regulations to guide the development of AI. On October 30, 2023, US President Biden signed an Executive Order on the Safe, Secure, and Trustworthy Development and Use of Artificial Intelligence. The order emphasizes the need for AI to be developed in a manner that respects civil rights, privacy and human values.
Shortly after, on December 8, 2023, the EU Parliament and Council reached a provisional agreement on The EU AI Act which aims to create a legal framework for trustworthy AI – addressing issues such as transparency, accountability and bias. It also introduces a set of requirements for AI systems that are considered high-risk, including those used in healthcare, transportation and law enforcement.
While new AI regulations are emerging, it's important not to overlook previously passed regulations that are still in effect, such as the General Data Protection Regulation (GDPR) and the Children's Online Privacy Protection Act (COPPA), which require developers to consider data privacy and consent at every stage of AI development.
Ensuring compliance
To comply with these orders and regulations, AI developers must ensure the transparency, ethical collection and protection of AI training data while upholding individual rights. Here are a few data-specific strategies that AI developers should implement to achieve this:
- Ensure AI training data is representative and diverse: Include a wide range of data sources that accurately represent the diverse user groups that will interact with your AI system to avoid biased or discriminatory outcomes.
- Implement data privacy and security measures: Protecting data, especially sensitive data, is paramount. Implement robust security measures to safeguard personal information and ensure compliance with data protection regulations.
- Provide data transparency: Be transparent about your data collection process to establish trust and credibility in your AI. Users should clearly understand how their data is collected, used and stored.
- Implement data governance: Develop and put into practice data governance policies and procedures that provide a framework for data management. They should cover data quality, access, retention and sharing, to maintain data consistency, accuracy and integrity.
- Establish mechanisms to address data incidents: Implement a clear and well-defined process to effectively handle data breaches and incidents, including incident response plans, communication protocols and procedures for notifying affected individuals or authorities.
- Educate employees: Provide adequate employee training on data privacy, ethics and responsible data handling practices to ensure everybody understands their role and responsibilities in protecting data.
- Engage with stakeholders: Regularly gather feedback from individuals whose data is being processed – including customers and relevant communities – to address their concerns, incorporate diverse perspectives into data practices and promote transparency, accountability and responsiveness in data handling.
- Continuously monitor and evaluate data practices: Conduct regular data audits and assessments to identify emerging data risks, address evolving data challenges and ensure ongoing compliance with ethical and regulatory standards, including staying up to date on emerging technologies, best practices and regulations.
- Incorporate ethical principles during development: Integrate ethical considerations such as fairness, non-discrimination and human oversight into your development process to avoid perpetuating biases or discriminatory outcomes and ensure the responsible and ethical use of data.
Special considerations
With new regulations being put into place, it's important to recognize that some previous, commonly used data practices can now expose developers to potential legal ramifications.
- Web scraping: While the automated scraping of data from the internet can be useful for quickly gathering large quantities of data, it often bypasses consent or permissions – directly conflicting with data privacy and copyright regulations.
- Social media datasets: The use of data from social media platforms to train AI poses significant privacy and consent issues. Those platforms often house personal and sensitive information, therefore using social media datasets without having secured explicit, informed consent from social media users can violate privacy laws and damage trust.
- Off-the-shelf datasets: AI developers must exercise due diligence, conduct thorough audits and understand how off-the-shelf datasets were collected and processed to ensure compliance with AI regulations.
- Explainability: Under the EU AI Act, users have a right to understand how a decision was made by an AI system, posing a challenge for existing complex, black box machine learning models. Ensuring interpretability and transparency during AI development, data preparation, training and deployment is no longer optional.
Harness the transformational power of AI responsibly
AI advancements continue to unlock possibilities and change our world in unprecedented and unexpected ways. However, ensuring AI is trustworthy is critical to responsibly harnessing its potential.
Regulatory compliance helps ensure the safety, privacy and rights of users, while building a firm foundation of trust. By prioritizing diversity, privacy, ethics and transparency when preparing AI training data, you can ensure your AI is trustworthy and beneficial to all.
Planning a generative AI project? Download our generative AI decision roadmap to understand key decisions you should make upfront to ensure project success.