Budgeting for your generative AI training project
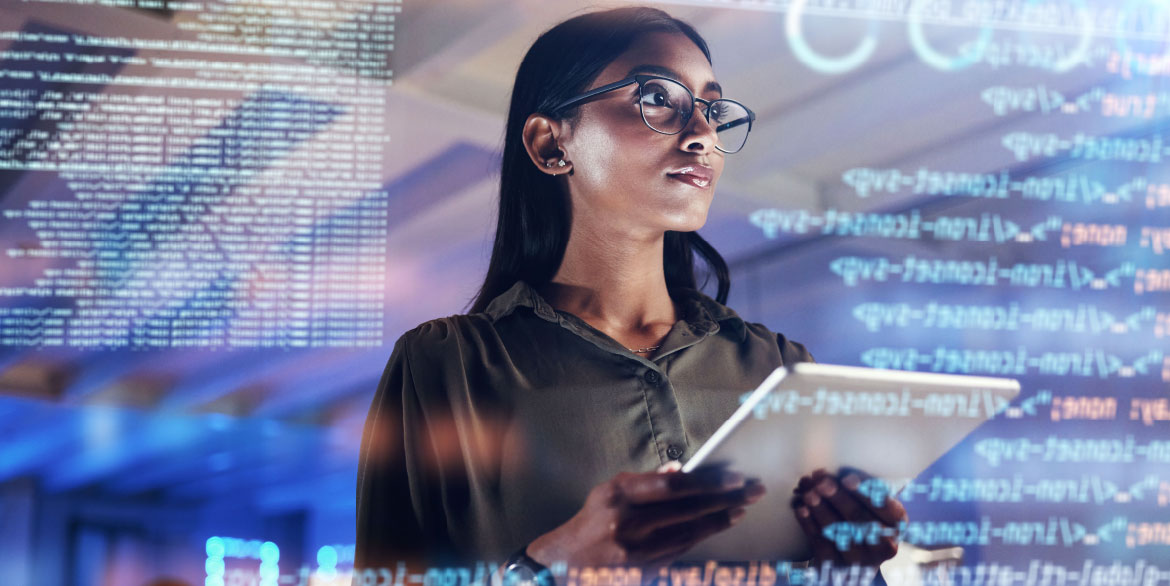
Artificial intelligence (AI) is only as good as the data it’s trained on. Put bluntly – garbage in, garbage out. To ensure more accurate, immersive and engaging AI experiences, generative AI engines must be trained on large volumes of high-quality data. But preparing the AI data you need to train your machine learning (ML) model is a monumental task that can consume up to 80% of AI project time, leaving little time to focus on developing, deploying and evaluating your AI applications. One possible solution? Working with the right AI data partner to deliver the exact data you need to train and fine-tune your generative AI.
But how much does AI data cost?
AI data vendors take different approaches to pricing AI training data. Some vendors price hourly based on actual time spent preparing the data, some price based on the number of data points delivered, while others price based on productivity considering the time it takes to complete each data task and the total number of tasks required.
Regardless of pricing approach, the cost of AI data ultimately depends on three key components:
- People
- Productivity
- Process
People
- Number of resources – Do you require vast volumes of data to train or fine-tune your generative AI application? If so, you should plan to engage a larger pool of data workers on your project. This is often a requirement if you need to improve a particular generative AI tool’s breadth of knowledge. It is also important to consider each and every workflow that may be required and their impact on the number of resources needed.
- Geographic, demographic, sociographic, or physiologic requirements – do you need participants to have specific features e.g. come from certain countries or regions, belong to a particular age range or ethnicity, speak specific languages or dialects with certain accents, or have a particular skin tone etc.? You should also consider whether the requirements of your generative AI go beyond language to incorporate culturally nuanced, locale-specific requirements such as an understanding of local culture, politics, destinations, etc., which varies between locations. And when it comes to language, though your generative AI may be multilingual, training and fine-tuning it often requires work to be completed in a single language by monolingual resources, as opposed to multilingual resources working with a source and target language. Therefore, it’s a good idea to consider the different hourly wages associated with the specific locales covered by your generative AI.
- Specialized skills – do your generative AI training or fine-tuning tasks require resources to have specialized knowledge such as multilingual skills, computer programming abilities, legal expertise, medical qualifications, specific hobbies, etc., or can anyone perform the tasks? Consider the expertise or specialized knowledge that may be required to successfully perform the tasks you need and the typical hourly pay rate for that expertise. For instance, doctors will demand a higher wage than creative writers. Also consider which tasks resources can be trained to perform vs. which tasks require specific expertise that cannot easily be taught.
Productivity
- Data type – what type of data e.g. text, audio/speech, image, or video, is required for generative AI training or testing? What data format(s) and file type(s) will be needed? Is there any additional context that may be required or helpful to resources in successfully completing their tasks?
- Task objective – does the task require resources to spend time researching or brainstorming? Oftentimes subjective tasks require additional time for resources to think and evaluate.
- Number of steps per task – how many different steps are required to complete one task? In some cases, one task may involve multiple steps, for example, rating your AI’s response time, evaluating the clarity of its answer, and verifying the factual accuracy of its output. Other tasks may only require resources to complete one step such as validating terminology usage.
- Time between tasks – Although the time between tasks may seem negligible, it does add up. Consider tools that could be used to improve resource efficiency vs. employing manual processes.
Process
- Training – do resources require training to successfully complete tasks? Consider not only project-specific training, but also general AI training that may be required for resources or domain experts who have never worked in an AI workflow before. Getting resources up to speed on 150-page guidelines will take significantly longer than 5-page guidelines. That’s not to say that 150-page guidelines may not be required for your project – only that it will take longer to train resources to successfully complete tasks and that additional training time must be factored into your budget.
- Tooling – what tools are being used and how much more efficient do they make your resources? Has quality assurance (QA) functionality been integrated into your tools to provide your AI data partner visibility into data quality KPIs? If not, additional upfront training will be required to ensure resources meet required quality thresholds without the benefit of a typical QA process which will also impact budget.
- Objectionable data – will resources be required to view objectionable data or content e.g. graphic violence, explicit language, etc.? If so, additional support will be required to ensure the wellness of resources working on your project, which comes at a cost. Additional resources are typically recruited for these types of projects to decrease the amount of time each resource must spend working with objectionable data. And with additional resources comes additional cost.